Example of Sequences in S-I
Rreasonable observation and occlusion properties are preserved in these sequences. This preservation ensures that these sequences inherit more realistic spatial relationships
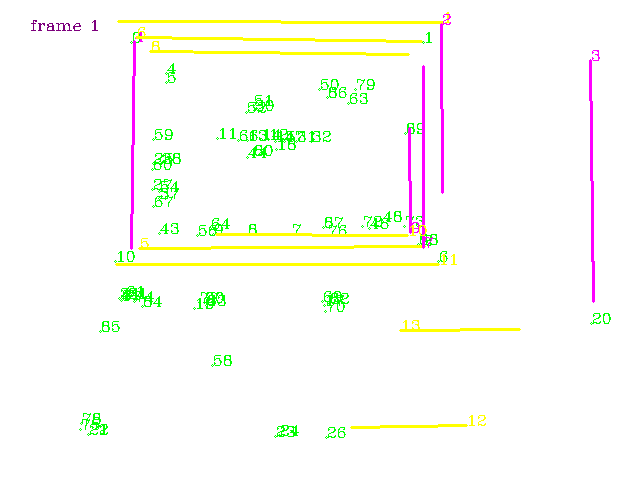
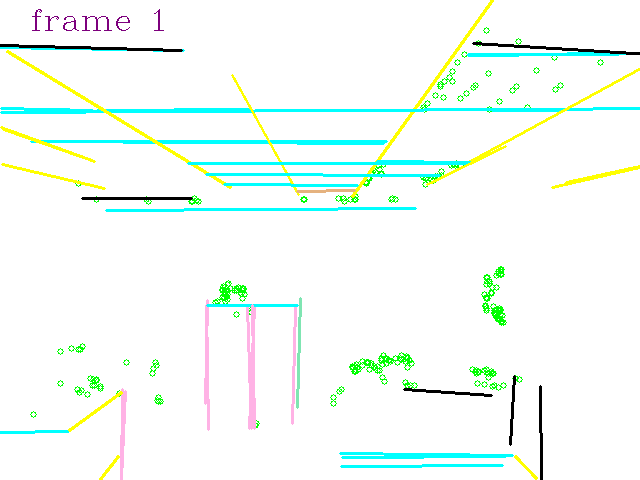
We present Open-Structure, a novel benchmark dataset for evaluating visual odometry and SLAM methods. Compared to existing public datasets that primarily offer raw images, Open-Structure provides direct access to point and line measurements, correspondences, structural associations, and co-visibility factor graphs, which can be fed to various stages of SLAM pipelines to mitigate the impact of data preprocessing modules in ablation experiments.
The dataset comprises two distinct types of sequences from the perspective of scenarios. The first type maintains reasonable observation and occlusion relationships, as these critical elements are extracted from public image-based sequences using our dataset generator. In contrast, the second type consists of carefully designed simulation sequences that enhance dataset diversity by introducing a wide range of trajectories and observations.
Furthermore, a baseline is proposed using our dataset to evaluate widely used modules, including camera pose tracking, parametrization, and factor graph optimization, within SLAM systems. By evaluating these state-of-the-art algorithms across different scenarios, we discern each module's strengths and weaknesses in the context of camera tracking and optimization processes.
Rreasonable observation and occlusion properties are preserved in these sequences. This preservation ensures that these sequences inherit more realistic spatial relationships
Simulated sequences enhance the dataset's diversity by introducing various carefully designed trajectories and scenes.
Instead of raw images, Open-Structure provides point measurements, line measurements, point landmarks, line landmarks, correspondences, and structural 3D lines to the community. This hot-swappable data format allows for flexible, effective, and fair evaluation of SLAM system modules.
ICL-NUIM
Open-Structure
office0: img | observation | initial factor graph | | livingroom0: img | observation | initial factor graph | | office1: img | observation | initial factor graph | | livingroom1: img | observation | initial factor graph | |
![]() |
![]() |
![]() |
![]() |
office0: img | observation | initial factor graph | | livingroom0: img | observation | initial factor graph | | office1: img | observation | initial factor graph | | livingroom1: img | observation | initial factor graph | |
![]() |
![]() |
![]() |
![]() |
office0: img | observation | initial factor graph | | livingroom0: img | observation | initial factor graph | | office1: img | observation | initial factor graph | | livingroom1: img | observation | initial factor graph | |
![]() |
![]() |
![]() |
![]() |
str_tex_near: img | observation | initial factor graph | | carwelding: img | observation | initial factor graph | | hospital: img | observation | initial factor graph | | japanesealley: img | observation | initial factor graph | |
![]() |
![]() |
![]() |
![]() |
box1: img | observation | initial factor graph | | sphere1: img | observation | initial factor graph | | corridor1: img | observation | initial factor graph | |
![]() |
![]() |
![]() |
box2: img | observation | initial factor graph | | sphere2: img | observation | initial factor graph | | corridor2: img | observation | initial factor graph | |
![]() |
![]() |
![]() |
@article{li2024open,
title={Open-Structure: Structural Benchmark Dataset for SLAM Algorithms},
author={Li, Yanyan and Guo, Zhao and Yang, Ze and Sun, Yanbiao and Zhao, Liang and Tombari, Federico},
journal={IEEE Robotics and Automation Letters},
year={2024},
publisher={IEEE}}